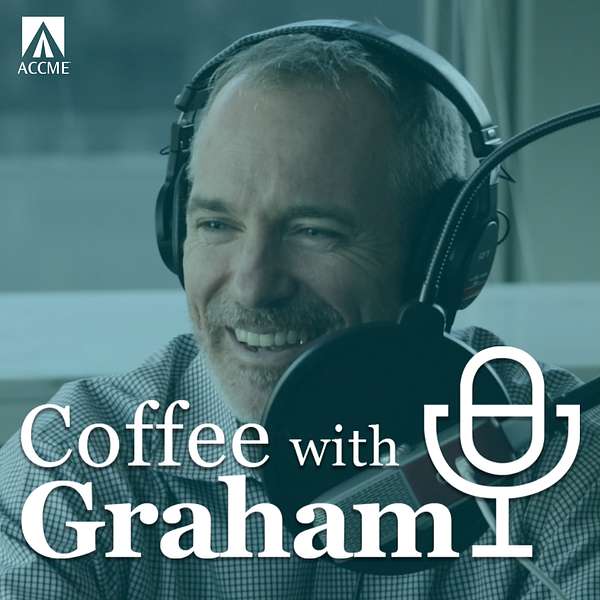
Coffee with Graham
Coffee with Graham
The Future of Artificial Intelligence in Healthcare
Dr. Graham McMahon talks to Dr. Peter Clardy and Dr. Paul Jhun from Google about the rise of artificial intelligence and how its use in the world of medicine can benefit continuing education and patient care.
00;00;01;00 - 00;00;37;25
Mimi Sang
Welcome to Coffee with Graham, a podcast brought to you by the Accreditation Council for Continuing Medical Education. I'm your host, Mimi Sang, and I'll be joined by ACCME President and CEO Dr. Graham McMahon as we discuss important topics brewing in the world of health care and continuing education. For this episode, we're joined by Dr. Paul Jun and Dr. Peter Clardy from Google as we discuss the rise of artificial intelligence and how it's use in the world of medicine could benefit continuing education and patient care. So grab yourself a cup of coffee or tea and join us for the discussion.
00;00;37;28 - 00;00;55;12
Graham McMahon
Hello, everybody. This is Graham McMahon here. I'm an endocrinologist and educator, President here at ACCME and delighted to be joined by two colleagues to explore the future of artificial intelligence, not just in health care but also in continuing education. Maybe, Peter, I could have you introduce yourself first.
00;00;55;15 - 00;01;48;21
Peter Clardy
Fantastic. Thanks, Graham. My name's Pete Clardy. I'm a pulmonary and critical care physician by training. My background is that I've spent about 20 years in the practice of critical care and was involved in both education at a medical student resident and continuing medical education level prior to joining Google at the end of 2021. My role at Google is focused on working with teams at Google, developing new technologies for providers in the health care space, and we've looked primarily at opportunities for using search and summarization over medical records. Increasingly, we're thinking about how artificial intelligence and generative AI impacts the space in both the way care is provided and the way we think about educating health professionals.
00;01;48;24 - 00;01;54;15
Graham McMahon
We're also delighted to be joined by one of Peter's colleagues at Google, Paul.
00;01;54;17 - 00;02;15;07
Paul Jhun
Hi, thanks for having us on. My name is Paul Jhun. I am an emergency medicine physician by training, and my role at Google really lies at the intersection of health professions, education, and technology and so it's quite relevant, if you will, to this contribution that we're about to have with regard to the future of artificial intelligence in health care. So happy to be here.
00;02;15;14 - 00;02;36;14
Mimi Sang
Thank you. I'd like to start our conversation by giving us a little background on artificial intelligence or A.I. So we hear more and more about artificial intelligence. But it's not always easy to understand what that term refers to and what that means. So, Paul, can you explain A.I. in the most basic sense for our listeners?
00;02;36;17 - 00;04;59;14
Paul Jhun
There's certainly been quite an ongoing explosion of conversation around AI or artificial intelligence, particularly in the field of medicine. And if I had to guess, most of us health professionals don't have time or haven't had the time to even really start digging into what artificial intelligence or A.I. means. But it's a lot of terminology and concepts have been thrown around. And so I thought that maybe it might be helpful to just hit some of the highlights because it's quite a large topic and we'll start broad and then narrow it down to the particular conversation we're going to have today about large language models, I think more specifically, the top of mind for a lot of us. So starting off broadly about artificial intelligence or AI, many would describe AI as a field of computer science, and it really refers to where computer systems can do or perform tasks that normally would require human intelligence. So common things you may see are like movie recommendations after you've seen a movie or customer support related types of assistance. But there are lots of different ways to categorize AI itself. And one of the ways to categorize it would be through the way that computer systems learn. So for instance, if we took traditional programming, which is more rules-based, let me use an example chess game. You program how a pond would move, how a rook would move, or how a knight would move. The rules of the game. And that's how traditional programming is. That's one way a computer system can learn. But then there's another way where, let's say you fed the computer system lots of data, and through that the computer system learns patterns, probabilities, creates statistical models. And so using that chess game example, instead of programming in the way that the rook moves or the knight moves, you're going to feed that computer system lots of different games of how they've been played appropriately. And it's learning the rules of the game because of the patterns and probabilities and the statistical modeling. So that concept of learning is machine learning. Let's say we have a bunch of pictures of cats and dogs. Okay? The goal is to identify the cat's picture of the cat. So traditional program would be you're going to put a lot of different characteristics of cats. So I don't know if you can tell me some characteristics of cats, maybe distinguishing ones from dogs.
00;04;59;17 - 00;05;02;09
Graham McMahon
Whiskers, pointy ears, pink nose.
00;05;02;12 - 00;05;17;28
Paul Jhun
There you go. Right. Or like maybe even the vertical slit-like pupils, right. I mean, like, those are the characteristics, those are the rules that computer system would then learn to be able to distinguish cats from dogs. If we're going to go the machine learning route, what would you think would be a way to train the computer system?
00;05;18;00 - 00;05;30;26
Graham McMahon
Obviously feed 100,000 pictures of cats and then 100,000 pictures dogs and let it work out the form, the features, the characteristics that allow it to make a statistical inference about the two.
00;05;30;28 - 00;06;46;12
Paul Jhun
Exactly. That's what machine learning is. And then you can dig a little bit deeper. You might have heard the term deep learning or neural networks, but that really is as the patterns get a lot more complex, as the statistical modeling techniques become a lot more nuanced and very layered, deep learning is a subset of machine learning. But taking one step back, like what we talked about was classifying or sorting different types of data, so that's a technique that is discriminative. So the computer system is learning patterns and probabilities and deriving or creating these statistical models to sort out a cat versus a dog. But what if you said we have these patterns of statistical models rather than sorting things out, let's generate and predict something new. So this is where we get into generative AI, right? So now we're actually creating new data based on the patterns and probabilities we've learned. And then when you talk about specific to language, that is where you get generative language models. And that leads us to the conversation mostly about large language models today, where large really is a reference to just a large amount of data and a large amount of hyper parameters or settings to these models that help tune and weight how we're going to be generating new data. So that's AI in the most basic sense.
00;06;46;14 - 00;06;56;05
Mimi Sang
Thank you very much for that amazing example. Could you please walk us through the critical elements of AI just to round out this intro session?
00;06;56;07 - 00;07;51;28
Paul Jhun
Yeah, sure. So I think that there's a lot of considerations when you talk about, let's say specifically the two LLMs. There are a lot of things that you have to keep in mind. But for the sake of time, I think hitting like three critical areas will be really important, I think, for all of us to just have a basic conceptual understanding on those three things are the importance of temperature, the importance of choosing the right model, and then lastly, the importance of prompt design. So I'll start off with temperature. Taking one big step back. I think we're all familiar with autocomplete, right? Like when you type into the search engine, you start typing a word and it will suggest how to complete that word or how to complete the phrase right alarms. In a sense, a different way of thinking about it is a really sophisticated autocomplete. Right? So let me give you an example and how this relates to temperature. Let's try this. So I'm going to come up with example. The bank robber stole. Fill in the blank, please.
00;07;52;00 - 00;07;52;20
Graham McMahon
The money?
00;07;52;22 - 00;08;04;26
Paul Jhun
Sure. Okay. Thank our money. How about we'll say money, category jewelry, watches, something like that. Right. It's really interesting how you came up with money so quickly. Why did you say money?
00;08;04;26 - 00;08;18;20
Graham McMahon
Well, that's what I associate a bank with more than a a locker of stored goods and personal items. I think of it as a repository for money. And most of the transactions I think about are related to money transactions at the bank.
00;08;18;22 - 00;09;24;00
Paul Jhun
Right. Because a robber could steal a bicycle, could steal antiques, could steal a car, right? I mean, like, there's so many things a robber could steal, but it was that bank. So attention to context is so important to understand what you decided to fill in the blank with a clinical correlation can also be like differential diagnoses, right? Because you have a certain sense of pretest probabilities. If I said, for instance, Graham like chest pain, I'm sure you have differential diagnosis, but that might change if I said, what if it's acute chest pain or a 19-year-old with acute chest pain versus a 75-year-old with acute chest pain, all of a sudden your probability is changed. So there is a setting on alarms called temperature where it ranges from 0 to 1, but really it can go to any positive number where the closer you are to zero, the more predictable, the more accurate or the more probable the output is going to be versus the more close to one or above and beyond, the less predictable, the less probable.
00;09;24;00 - 00;09;41;14
Paul Jhun
And also, I guess you could say the quote unquote, more creative the output would be. And so knowing what your model's temperature setting is, informs the kind of output that you should be expecting, right. So, Graham, if you wanted to create a poem, would you want a higher or lower temperature?
00;09;41;14 - 00;09;43;12
Graham McMahon
Always higher. Super creative.
00;09;43;14 - 00;09;50;05
Paul Jhun
Right, versus let's say you want a translation of a technical document, like would you want a lower or higher temperature on that?
00;09;50;10 - 00;09;55;13
Graham McMahon
Yeah, obviously a lower number. You don't want creativity and a technical document; that's not helpful.
00;09;55;16 - 00;12;19;04
Paul Jhun
Right! So intuitively that makes a lot of sense. But in my conversations with a lot of healthcare professionals, it's like when you start lifting up the hood and seeing how the engine is built, it's like, Oh, there are things that you can tweak in some cases to be able to adjust to get the output that you're desiring. So then the second issue, we talked about temperature, now we’ll talk about choosing the right model at a high, high level. There are foundational models and then there are fine-tuned models, foundational models in the sense you could think of it like a general skills, all purpose. It's a general skill about how to generate text, answer questions, summarize, and then they're fine to models where you want to focus in on a more specific type of task or specific knowledge domain where you're actually training the foundation model to a very specific use case. And the analogy I can use is like a screwdriver, right? You buy a screwdriver, it has a handle, it has a shaft and it has a flat head tip by default. And you could use that to screw in like a cross or like a hex. I mean, it can kind of work, but really, you want to switch out that tip to the appropriate use case for the screw. And that kind of makes a lot of sense. So that's making sure you're choosing the right model for the use case is important to be aware of. And then the last part is about the prompt design. So temperature, choosing the right model, and importance of prompt design in limbs, the output really significantly depends on the input that you put in. So that's called a prompt and the clinical corollary can use is whenever you get a patient history. I'm sure we all remember when we were in training and we asked the patient a set of questions in a history and then all of a sudden the attending asks the questions and they got a totally different answer. And it's like, what? Right? And so in many ways, how you ask and what you ask will determine the answer that you get. And so from that perspective, prompt design is really like an art in a science where you want to be specific, you want to provide some context or like examples maybe, and you really got to experiment. You got to practice that skill in order, develop it to understand. Don't just give up after the first try. Well, what if I ask, what is diabetes? Well, are you asking for a patient or are you asking for professional? Or maybe your question wasn't about that. It's about treatment of diabetes. Or maybe it's even more specific about SGLT2 inhibitors, right. And how to actually dose it. It's like that level of specificity and using examples of what you want that output to be. Prompt design is a very important concept. So those are the three things I think at a conceptual level are important for those to understand.
00;12;19;06 - 00;12;40;27
Mimi Sang
Great. Thank you. Now that we have our best understanding, we can get into our larger conversation here, more about AI's impacts on clinicians and their patients. So what would you say, Peter, to start out, would you think is AI's impact on clinicians and their patients?
00;12;41;00 - 00;18;50;07
Peter Clardy
Thanks very much. It's a great question and I would sort of place us a little bit in a historical context of of where we are right now in a journey to integrating AI in clinical practice. And I think to Paul's point, there has been a sort of decades-long evolution of AI technology that in the past several years has really undergone an inflection point, and that represents a transition from some of the more historical models that we might use for annotation to these more generative models and, and the implications for what that may imply for health delivery, I think are really in the earliest days of understanding. But at a high level, I think most clinicians would recognize phases through which their care practice moves in the transaction with an individual patient. We spend a lot of time assessing what gets us up to right now. What are all all of this kind of universe of data that surrounds the patient and increasingly recognizing that is EHR data, often from multiple EHRs, but also inputs from wearables and other devices. And I think one of the challenges that clinicians feel now relates to how do we assess patients in an era where we both are faced with profound data fragmentation, sort of only seeing a piece of the picture. But if we had all of that data together, we're faced increasingly with problems of data overload. And when you think about that assessment process, when you're seeing a new patient, what do we do? We browse by data type, we look at notes, we look at labs, we look at vitals, we look at reports, and we integrate across all of that data to create a disease representation of what's going on with this condition or with this patient. So one way to think about the promise of AI in assessment would be are there better ways of organizing this complicated, messy, diffuse universe of information that surrounds a patient that help with things like clinical summarization. And so those are some areas on the assessment side where generative models might be able to reduce some of the complexity of integrating across all of this complex data to create more detailed but also more immediately accessible representations of patient disease state. The other sort of aspects, right, as you sort of think about that clinical transaction, one big area relates to decision-making. How can we make sure once we know what's going on with the patient, that our thinking is clear. Are we doing the basic things correctly? Are we sort of applying logic in the right way? This is an area honestly where I think we are entering with great humility and I think an area where we probably have a lot to learn about the human-AI interaction around some of those decision making processes. So I'm going to say in some ways that to me is the the final area to enter into. And though it has great opportunity, it also comes with a certain sort of risk. The third phase is the workflow phase, right? So we generate output based on our assessments of all of this data. And in some cases that's a procedure, an operation, or a prescription. But in most cases that is a document of some sort: a note, a referral, a letter, a communication. And here these generative models have really remarkable ability to sort of assist with certain kinds of of workflow assistance in terms of generating text, which could be proposed as a draft, reviewed by a provider, and then finalized. So I think from my perspective, there are opportunities along this continuum of assessment, decision-making and workflow support where AI could be usefully applied. I think that the sort of the next question is, well, why has that not happened? And in some ways I think here there are some important considerations that we can talk about, about sort of why I see us as sort of in the early innings of the long game when we think about the safe and effective application of generative, AI to clinical decision making and to clinical care. This is in some ways a first in human history sort of problem, right? These are new systems applied to new sort of use cases. And I think that while we have a lot of ideas about sort of how assessment should look for tools like this, it is really different from assessing other sorts of technology. And so I think we can maybe go a few levels deeper on some of the complexities. But in the same way that when I was a kid, the advent of cheap and easily available handheld calculators was a game changer in terms of how we thought about how we would learn math and how we would be educated. I see the use of bedside ultrasound, which changed practice in critical care, but required sort of effortful resourcing to learn about the technology before using it with a patient or in a clinical context. I anticipate something similar where really the first set of challenges is about teaching providers. What are the opportunities and what are the limitations of this new technology. There's a famous quote that we've bounced around that I think bears some reflection, which is that you want to shape your tools before your tools shape you. And I think that that's a little bit of where we are with the integration of of AI into clinical practice, which basically means that the first step is really in understanding what these models do and what they don't do. Some of the ways that they can both be helpful but also risky. And that's probably where the conversation begins.
00;18;50;10 - 00;18;58;27
Mimi Sang
Graham, Would you like to speak on any difficulties that you think clinicians might experience related to their patients using AI?
00;18;59;00 - 00;23;16;05
Graham McMahon
All sorts of things to talk about here. But the first is some doctors think that they're going to be out of a job because the AI is going to do it for them. And if you take the example of things like closed loop insulin delivery systems in my world, in diabetes, that has just elaborated and clarified the extraordinary importance of expertise in managing increasingly complicated systems. And that is going to be true for technology here too. Technology is only going to make our jobs both more complicated, but also more interesting and allow us to do things we've never been able to do before because AI can do things with our patients between historical visits rather than, you know, just at those sort of single encounters. So the first thing is to reassure clinicians that nobody's going to be out of a job any time soon. These systems are here to stay and fighting them is almost pointless. We have to find ways, as Paul said and Peter said, of engaging with them, controlling them, understanding them, utilizing them in a way that helps patients. But patients have to have access to these technologies, of course, too. And a key challenge that we have in thinking about the implementation of health care through generative AI and these types of processes means that we have to find ways of incorporating into practice that is not so disruptive and that actually improves efficiency. And that's a very hard undertaking to implement vast new technological automations and systems without elaborating the complexity of the implementation by the patient, the pharmacy, the surgery center, the doctor themselves. All of that is a challenge. I think the third thing is that we do, though, have to change the way in which we operate. If you think about the capacities of AI that Peter was just talking about, the way it can take multiple different data sets and incorporate them into new observations about a patient, that is extraordinarily powerful. And in our world, for example, if you think about insulin delivery, you know, a rules-based program would say the patient's blood sugar is high. If a patient's blood sugar is high, tell the pump to give more insulin and we would have some rules about how much insulin to give. That's a rules-based program and it's lovely to have, it's very powerful, it's very helpful for patients, but it is not generative AI. And I would say, hey, Graham's glucose level around lunchtime tends to respond a lot more quickly to insulin, particularly when he has popcorn, and he's just told me he's having popcorn. As a result, I'm going to make a different adjustment to the insulin I'm going to deliver for Graham at his lunchtime compared to what I might do for Peter. And that is a very different approach to using technology, not better rules-based systems, but a totally different set of expectations and technologies for how these operate. You incorporate that to say, let's mix medical records between a patient and their parents. For example. You can take implications that are in a parent's medical record, apply them to this individual patient. This young woman has just become pregnant; her mother has hypothyroidism. Maybe she should be screened for hypothyroidism and you wouldn't be able to generate those types of observations unless you had mixes of the data that were made, allocations that are new and potentially generative. I think also this idea that we'll be prescribing algorithms of care, not a rule, essentially “give this patient ten milligrams of lisinopril” won't be the approach anymore. It'll be: “deliver algorithm 10A to this patient with hypertension” and let the AI incorporate the patient's home blood pressure measurements and prescribe first increasing dose of lisinopril, then add on the hydrochlorothiazide, then add on the amlodipine, what have you, as patients care doesn't respond to initial interventions. If you particularly incorporate that with home-based observations, let's say urine analysis, you know, you can actually start to make algorithms of care that clinicians can essentially prescribe, and that is a systematic change in the way in which we implement care quality and will require the profession to adjust to a totally new way of handling medicine and healthcare in the future.
00;23;16;07 - 00;25;10;10
Peter Clardy
There's so much, Graham, in what you shared that I am strongly aligned with and really appreciate that as a as a summary. One thing that I wanted to to just kind of bright light a little bit because I think you've done a really nice job of kind of drawing it out is that sometimes there's a conception that these new powerful models are in and of themselves a solution to an unmet need or a problem. And in fact, it really requires very careful implementation at a use case level that really requires understanding sort of the details of what the care pathway or what ideal care should look like. But I also wanted to flag one thing that you mentioned and I think is sort of foundational to the way we are thinking about a lot of these efforts, which is how can you make these tools assistive and not intrusive? How can you make them useful but not proscriptive in that sort of interaction between the human and the AI? So I think part of that always requires a human in the loop, you know, that we wouldn't propose that the read of the x-ray generated by the AI is the final read, but rather these tools may help with workflow to quickly flag things that look abnormal or to help with overreading or recognizing things that might be missed. And I think that for me as a clinician in an era of rapid change is something that's important to sort of call back to, which is that human in the loop is really sort of a foundational principle of of how to think about these tools. And so as you go from a model to an actual product or a use case, I think it's it's helpful to think what's going to be assistive to this provider, what's going to allow them to have the right level of engagement with the data, but what's not going to sort of steamroll human expertise in the implementation process.
00;25;10;13 - 00;25;57;03
Graham McMahon
Yeah, so, so much there are, Peter, in terms of how it's going to affect our practices, but the ways in which it can engage patients in a different way at their right reading level, in a communication style that's best for them, and using a technology that they actually utilize and that looks at engagement patterns to customize messages or ways of behavior modification and support that are going to change patients. But the ways in which, you know, our interactions with patients even, who gets to see the doctor versus who gets to see the nurse looking at the context of care and how a patient is doing, maybe that patient is better off spending their time with a nutritionist as compared to the nurse or the doctor, for example. So these types of imputations you can make from these rich datasets are potentially very powerful, maybe even transformative for the profession.
00;25;57;05 - 00;26;33;05
Peter Clardy
Agree. And I think you highlighted something else which which bears consideration, which is that a lot of what you see if you're analyzing an EHR or sort of the standard parts of the medical record are very patient centered. But as you sort of work out further to data about family, about community, about geography, about availability of support services, medications, food. If you think about sort of the implications of climate we have now really rich and in some cases publicly available datasets that may help us bring new kinds of understanding to to just what you're describing.
00;26;33;07 - 00;26;48;19
Mimi Sang
I know we've touched on this a little bit, discussing everything going on with the risks and challenges for clinicians. But Graham, do you have anything else you'd like to add on risks and challenges of implementing AI and medicine as a whole?
00;26;48;22 - 00;30;02;07
Graham McMahon
Wow, that's a huge topic because there are so many risks and challenges for novelty and innovation in health care, particularly when it potentially introduces meaningful risk for the patient. The first thing I would say is that we as humans often don't consider our own error rate and will unfairly judge the error rate of the technology when there's a single problem. And we have to acknowledge that at some point technology will be better than humans for a variety of decision-making in health care. Ultimately, as Peter said quite correctly, there's almost certainly going to be a human at the other end trying to make a final determination with the assistance of these technologies. But we have to acknowledge that humans are not infallible and human doctors make mistakes and make them with a degree of frequency that is sometimes going to be illuminated and elaborated by the availability of technology to help us. I think there are big issues, though, more fundamental even than accepting an error rate, and that is things like ethics in medicine. Ethics has suffused the profession since its inception, and ethics will need to infuse technology models too. And you already heard from Peter how complicated it is to introduce context into decision-making and the ethics of how we model design, how data sets will be incorporated into these systems. We've seen what major negative effects can occur if you incorporate data models that are at their basic level inequity or promote inequity or are not generalizable for the broader community, and that is seriously problematic. I think we have questions about autonomy and accountability when we think about AI. If I prescribe an algorithm and the patient does poorly, whose fault is that? Let's say the patient gets an overdose of insulin because the algorithm didn't work correctly. Is that my fault? Is that the models fault? Whose insurance plan is going to pick up that risk? And that's a major issue for us in the profession because we worry about harm for our patients and there has to be accountability and where that lands is is not yet determined. There is potential for misuse of technology both to restrict access to care, restrict costs, deliver, you know, say, marketing messages, using it to invade privacy to, you know, take advantage of the situation and the communication vehicles to advertise or other things to a patient that's risky. And then you have, you know, historical problems that we have related to privacy. And what are we going to do if we need access to this vast amount of information to make generative AI determinations? How are you going to control that sea of information to make it safely available to the models without making it even slightly available to those who would take advantage of it and misuse it? So not small undertakings and not small challenges for all of us as we think about this future. Lots of potential benefits, but also meaningful risks. And as a profession, we have to navigate those too, and control our engagement with these technologies and systems so that the public and the profession are beneficiaries of it and not victims.
00;30;02;10 - 00;32;44;16
Peter Clardy
I really appreciate your thoughtful reflection on that, Graham, and it really matches a lot of my own. There's one or two things that are even more specific to some of these generative AI models that are worth considering as well. You brought up the issue of bias and that when you sort of think about how these are very large foundations are trained the datasets over which they are sort of resourcing all of the insights that they bring, essentially this representation that those data sets in and of themselves have bias, whether that's the Internet or Wikipedia or even PubMed. And some of those biases are well recognized and some of those biases are really only now or in the future are going to be fully appreciated. So there are some challenges based on sort of what we're building with. There are also some really interesting properties of generative AI models, which include things like so-called emergent features. So these are capabilities that models have that were not explicitly trained. So things might be certain kinds of summarization where you've created a model that can summarize things, but you ask it to summarize it in the form of a sonnet, and you see an output that that actually reflects that oh, the AI is capable of both handling this information and manipulating the form in this way, which we didn't actually explicitly teach the model to do. There are also some challenges with these emergent type properties and a related issue with something called hallucination, where the and again, it's hard to speak about this without sounding a little bit anthropomorphic, but I understand the way that models work in terms of completing next token in a sequence of sort of wanting a certain kind of completion to a thought. And as a result the outputs are often quite fluent and sound very much the way a medical note or a provider might see. But you will see things in these outputs that are actually not factually accurate. And those sorts of challenges you can address to some degree with what Paul described by sort of turning down the temperature and really leaning in to factual accuracy. You can get around that to some degree by the way you tune these models. But I do think it's important to acknowledge that it’s still an area of concern and consideration as you think about the application of certain sorts of generative A.I. models, because there's sort of this flipside to emergent properties is this tendency to want to complete things, even if it's adding information that isn't present in the record. And obviously for certain kinds of creative outputs, that's fine. But in healthcare, that's really not.
00;32;44;19 - 00;33;03;29
Mimi Sang
And Graham, did you have any advice that you would want to share with health care professionals and educators who might feel a little unsure about utilizing AI in their practice? I know we've discussed some of these risks, but we also know that there are so many benefits to using AI. So what would you say to that grouping of people?
00;33;04;04 - 00;34;22;28
Graham McMahon
I would say educate yourself. Listen to Paul and help yourself understand what's going on in this environment and try and suspend your inevitable fear and worry about these systems for now. Learn about them, engage with them, test out the basic models that are available from a consumer perspective almost for fun. So you get a little more comfortable with the kinds of things it can do for you, even with a medical question. And be ready to be impressed and then wait for the trials that are inevitable as we determine how these models work and whether they work better than the standard algorithm of care, for example, based on our office visit. These are things that are going to have to be trialed out before they're implemented, and it's going to be a while before we have that type of information. But I think there's a lot of things you're going to see in things like electronic health records, in lab reports, for example, in the way in which we use home patient data being presented to us, that is going to increasingly use generative deductions from the material to help us understand and contextualize the information that's being presented. So it's not just a bunch of numbers, but a whole lot of information that helps us interpret the data or give us a heads up on our interpretation that technology is used.
00;34;23;00 - 00;34;25;10
Mimi Sang
And Paul, would you like to share?
00;34;25;12 - 00;35;45;19
Paul Jhun
Yeah We talk about the theme of education in AI first because it's foundational before we really talk about AI in education or health. And so there's kind of that sequence where in many ways there's a phrase that has been used, I don't know how to attribute it, but instead of artificial intelligence, augmented intelligence. And so in many ways it's really about augmentation and not automation. And the corollary that I think about is when clinical decision tools have been proposed, derived, and validated. Right? In many ways, the goal is to improve our clinical efficiency and outcomes. And what we need to think about is it's similar in the sense of how A.I. will be implemented. In many ways we are still in the driver's seat. What we need to recognize is that we are the ones using the tool, which means you need to understand how it works, why it was designed, when to use it, when to not use it. This is such an interesting opportunity where we talked about things like bias, where in a meta moment AI can also help service bias or service the gaps in our own knowledge in many ways. So like there are certainly wonderful opportunities and we can use this moment as its topic of the day and leverage the moment.
00;35;45;22 - 00;36;02;13
Mimi Sang
I know we've spent the beginning of this time talking about AI in general and talking about AI in the health care setting. And just to zoom in a little bit, how do we think AI could be used effectively in continuing education? Peter, would you like to start us off?
00;36;02;15 - 00;40;19;18
Peter Clardy
Yeah. So as an educator, this is something that we've really had an exciting time thinking about, and I would sort of say that there are lots of efforts that have really started to think about how generative AI can impact education at all levels. And there are tools that are increasingly available to sort of lower barriers to access. Graham mentioned ways to get started and sort of ways to think about how to incorporate generative AI into practice. And education is a really great early use case in some ways because the risks may be somewhat lower and it does allow individual folks to explore the technology a little bit on their own. There are a number of ways I think you can think about how an individual could use generative AI for their own personal learning and then how teams or groups might use generative AI for shared learning experiences in a sort of continuing education model. One of the things that these tools are very, very good at is summarization. So, for example, there are now fairly widely available tools that would allow you to generate a whole bunch of references on a particular topic and then create summaries or ask questions or essentially quiz your own knowledge of that information in a way that's going to be much more specific to your own needs or learning goals. For educators, there are also opportunities to use generative AI to create high-fidelity learning simulation scenarios. So one of the things in addition to summarization generative, I can be very good at kind of working from example. So if you provide a few very well-grounded and sort of physiologically reasonable examples of interactive case presentations using generative AI models, you can take that work of scaling up, which on a human basis is extremely time consuming and methodical to create a library of teaching cases where generative AI may allow that to happen at at a much greater rate and with greater fidelity as well, there are other ways to think about how generative AI might in the future create opportunities that help providers focus on areas of particular need. I think we've all had the experience of sort of going through review questions and maybe there are big chunks that are very familiar stuff that you see with great regularity that you don't need a deep dive on. But these things that they present very infrequently in clinical practice or have changed recently or are evolving in the literature where tools that would allow you to particularly see things that might be in your blind spot could be surfaced. And again, that's, I think, further down the road from where we are right now. But it's really exciting to think about some of those opportunities. The last one I'll leave with is one that I think is interesting. Just sort of think about in terms of the range of possibilities, which is you can think of a generative AI with a number of different personas. In other words, you might have the persona of a teacher where the learner would be able to ask questions and get summarized answers from the AI. You might also think of the AI as generating useful questions for the student and sort of be more the proctor for examination type questions. And you can even think about situations where you might take the AI and have that in the role of the patient so that you could look at certain kinds of interactions back and forth between a patient and a provider and use that as an opportunity to optimize for certain sort of outcomes. So I think that the opportunities in the education space for generative AI really cover a very, very wide range. And I think in some ways it's useful for people to consider where are the challenges, what are they doing now, how might these tools impact their ability to create either for themselves or for other folks compelling educational tools.
00;40;19;20 - 00;40;23;11
Mimi Sang
And Graham, do you have anything you'd like to share on that front?
00;40;23;14 - 00;45;34;13
Graham McMahon
Lots. It's such a fascinating area because human memory and learning as a physiological construct is pretty well understood and is modifiable through direct educational deployment and technology, and in particular, nuanced AI-based technology can be very effective at augmenting things like retention application and learning and quite profound ways. But the CE community as a whole is going to have to deal with AI and will be dealing with the AI in a variety of ways at five levels. You’ve got the accreditation system and how we're going to work with AI. You've got the educational provider, you've got the activities they produce, you've got the learner themselves, and then you've got the assessment and outcomes and the connection to learning outcomes related to all of those that are all modified and augmented by effective implementation of AI on an accreditation level, obviously making imputations on thousands of pages of information coming from an educational provider is much easier, as Peter said, for summarization, looking for a thematic analysis, looking for evaluation of errors, etc. in the accreditation file. That'll be easier for the technology than it will be for human review or in many cases, or at least serve indications of strengths and weaknesses in an application that will be very helpful to us. On the provider level, I think the opportunities in AI are much more substantial than most providers actually think because not just making I say a needs assessment but also making overall reusing or recompiling stored information that you already have recorded in segments, let's say there's three minutes of one of your one-hour lectures on or sessions on insulin delivery that's specific to carb counting for a patient with type one diabetes that suddenly is now available and acceptable in a very efficient way to a session specific to patients with type one diabetes. But on the activity level, banal things like recruiting best speakers based on generative AI's ability to source the best people who have the highest ratings nationally when they talk about diabetes, specifically on X or Y topic, those kinds of things are going to be available to educational planners and they'll be able to pull information from a variety of sources to help to compile activities that are going to make most sense. Similarly, just compiling an activity is going to be a new experience because different learners are going to be looking for a different experience of learning. So, for example, let's push on this example of a Type one diabetes session. Some might want to hear a podcast about it, some might want to do a tactical or psychomotor exercise related to programming an insulin pump, and some others may want to hear about it or see a video about some model. And an AI could take a piece of text or a demonstration, and we already know that that can be converted into a podcast, can be put into a slide context, converted into an interactive education experience. We’re getting to a point where you can auto-compile educational formats based on individual learners’ preference and convey the same type of learning experience. From the learner's perspective, just think of the ability for an AI system to auto generate the types of reminders, the repetitions, the reinforcements that are going to be right for that learner. What Paul needs is going to be different from what Graham and Peter and Mimi need. We all need different types of reinforcements on different areas. And if an AI system is able to start tracking what we know, what we need augmentation on, support on, reminders about, and time both the format and the delivery of those reminders to be ideal for my memory decay curve or Paul's or Peter's. Now you've got pretty sophisticated ways to augment human intelligence and competency in ways that are quite powerful and likely to be very relevant for the types of sophisticated learners our system tries to work with and finally, then you think about assessment and outcomes, and there the AI is incredibly powerful to try and reach into an EHR you know, and see has Paul changed his practice, have his patients done better? Do Peter's patients with asthma now have a reduced readmission rate because he's implemented a different practice around bronchodialators? You know, whatever the issues are, you can make assessments and outcomes compiled for you, but you can also auto-compile the very assessment that you want to use and it can be at the highest level because you can track individual behavior, not just what they say they might do or what they think they might do, but you can actually track what they have actually done. Those types of implications for continuing education are obviously extraordinary. They are really exciting. They're terrifying. And there's that mix of both awe and excitement and optimism and fear that surround this whole area. But as Peter said at the start of this conversation, education is an area that's extremely ripe for meaningful disruption, but also improvement by the sophistication of educational technology and generative AI in that space.
00;45;34;15 - 00;46;18;05
Paul Jhun
I appreciate how you framed the opportunities of impacting education. I just wanted to add another consideration, which is in the effort to improve learner outcomes, also accessibility. There's an opportunity with ISE specifically in improving equity and globalization in many ways where, for instance, we all are familiar with closed captioning and text to speech. But then what about images, and can you take an image into text, into speech or translation and assistance with limited language proficiency? So like those are certainly wonderful opportunities where we can reach larger audiences and also improve accessibility and fairness for our learners overall.
00;46;18;07 - 00;46;32;26
Mimi Sang
That's an excellent point. And with this wealth of opportunity that we have for continuing education, what changes do you think the accredited CE system may need to make in response to AI? Graham, would you like to start us off?
00;46;32;29 - 00;47;06;17
Graham McMahon
We have a lot of interesting and exciting work ahead of us, but it is work nonetheless because change is hard and disrupting the status quo is always interesting and difficult and tumultuous. But it's necessary and inevitable that we have to be on this journey. So I think all of us are going to, as a community, look at these areas from learner data provider information, how we work with information, how we make accreditation decisions. All of these things are up for discussion and debate. But the one thing we won't be doing is standing on ceremony and sticking with the status quo.
00;47;06;20 - 00;48;22;17
Peter Clardy
I think it's a really interesting set of questions around sort of what this means and even to sort of go a little bit more broadly than continuing education for providers, I think there are a number of things about the advent of certain types of generative AI that really call into question a lot of the sort of standard approach to things like plagiarism and authorship and a lot that that is really foundational to many different types of educational endeavor. I think some folks have suggested, and I would count myself sort of among this group, that the development of these systems really represents a sort of epistemic shift, meaning a fundamental shift in the way people interact with information that will require a fundamental change in the way certain sorts of information is shared, communicated, and taught. And so I think that there are a lot of details at the sort of individuals’ health care provider level. But I would sort of encourage us to think of it almost more generally as what are the challenges now to sort of education and ways of learning and teaching that we can adapt into a health care space, kind of learning from best practice in a world that's changing very, very quickly.
00;48;22;19 - 00;48;31;16
Mimi Sang
And now we've come to our final question: Where do we think that the future of AI in health care is headed? Paul, if you'd like to start as well, please go ahead.
00;48;31;18 - 00;48;51;06
Paul Jhun
I think that in the short term, what's really promising coming down the pipeline is multimodal. It's already in many ways present, which is instead of the large language models that we've been talking about, now we're talking about how do you ingest different types of media? So we're talking visual, audio. And so from that perspective, that is the most interesting to be on the lookout for.
00;48;51;09 - 00;50;52;25
Peter Clardy
Yeah, I would agree that Multimodal and the sort of evolution of both bigger and more powerful foundation models that are looking at things other than or in addition to language really will represent new opportunities for these emergent properties. I think that where I am excited to sort of think about the evolution of generative AI is maybe a little bit more tactical in the short run, which is to say we've developed models that can do a really incredible human or nearly human or even advanced human job on certain sorts of tasks like medical question answering. We have tools that can do really, really well on the medical boards, but life is not a series of boards questions. And so where I see the excitement in this space is to really look at the real-world use cases, the challenges that everyone is facing right now, and think about how these tools will really address the problems as we experience them today, as we continue to iterate forward on new opportunities. I really love, Graham, the way you describe a fundamental shift in how we think about what medical intervention looks like. It doesn't necessarily look like a single static prescription at a given point in time because of a single blood pressure, right? It's part of an algorithmic approach that will evolve in a very patient-specific way over time with checkpoints that are going to be a lot more frequent than your annual visit. That's to me sort of the long vision. But I think that really the exciting part is that every day we're seeing opportunities and short-term wins in this space that I think really are signposts for where we can develop over time. And just to sort of circle back to education as being a really unique sort of context and frame for us to think about these opportunities to develop and scale new technologies.
00;50;52;27 - 00;53;08;11
Graham McMahon
Yeah, I agree, Peter. It's a really exciting time. It's also a pretty frightening time. We as clinicians have never had, or very few of us have had any training in technology. We've never had really being able to prescribe or do medicine in the way that is likely to have us doing in the next few years. And shifting the profession away from the current standard of approach to medical care, particularly for chronic diseases, away to an algorithmic approach and one that’s AI-facilitated is going to be an implementation nightmare, but also an incredibly exciting transition for the profession to optimize the health of populations. And that's the opportunity and capacity our system has. I think it's going to be very challenging for medical professionals in particular as simpler and algorithmically delivered care does not need to involve the physician anymore and how that affects the types of patients the physician is now responsible for and their complexity is going to be a real challenge for us because I won't be seeing the patient who's on metformin and glipizide for their well-controlled diabetes anymore. You know that patient is going to be well taken care of by the computer or one of my colleagues, but I'll be seeing the patient who's got renal failure, abnormal liver tests, and who's not tolerating insulin and having severe hypoglycemia despite an algorithm doing its best to control the patient's blood sugar. And that's going to be challenging for me just from a cognitive load perspective as well as just the complexity of these types of patients I'm now going to be responsible for if computers are doing more for us, but fundamentally we as a profession are going to have to change and evolve as we've done so many times before. We'll continue to do so. And we're fortunate that not only is continuing education there to help us as a community make that evolution and make those steps forward, but it'll be part of the transition itself and we'll be working hard at ACCME to try and make sure that AI is a supportive, positive impact on our community and our systems of healthcare service delivery as much as we will be trying to take care that it doesn't affect the ethics, the integrity, the core value proposition of what it means to be a human being taking care of another human being in medicine.
00;53;08;14 - 00;53;27;07
Mimi Sang
Thank you very much, all three of you, for embarking on this complicated, interesting, engaging discussion as we start to think about artificial intelligence in the medical world. It's already here, so we just have to decide how we can best move forward. Thank you to all three of you, and I appreciate your time.